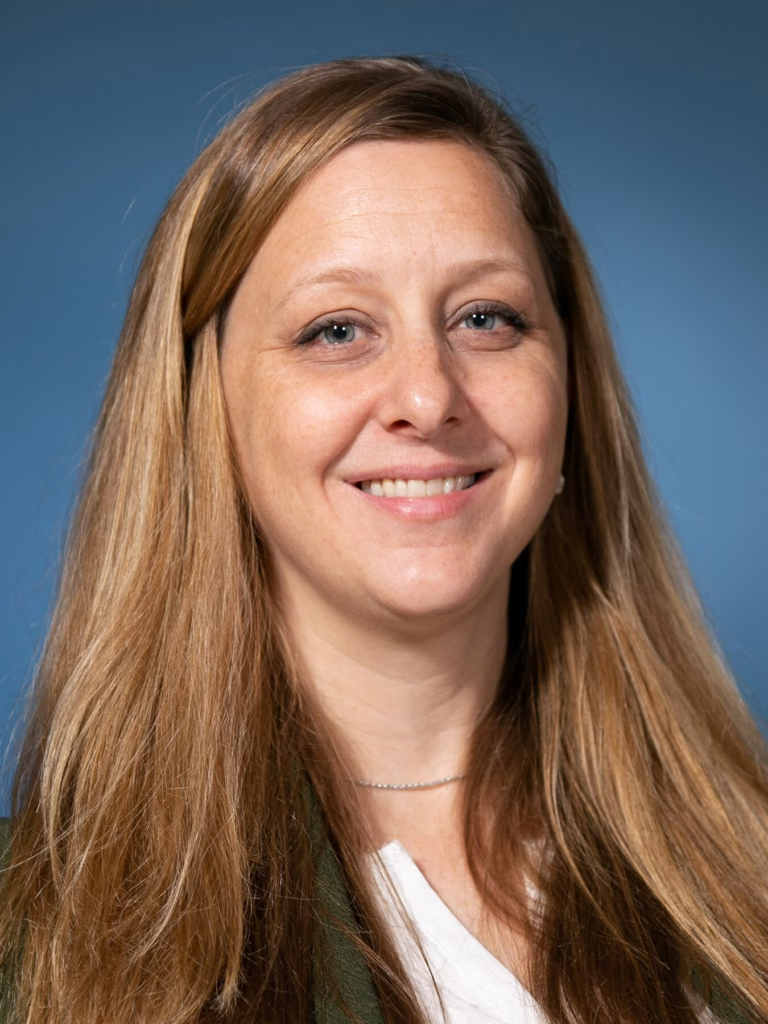
What is your role at UMass Memorial Health and how did you get involved with the Coalition for Health AI (CHAI)?
I’m Dr. Elisabeth Garwood, a musculoskeletal radiologist and Associate Chief Medical Informatics Officer for UMass Memorial Health. I joined CHAI in 2022 as a member of the safety working group. I was an editorial lead and wrote portions of the assurance standards guide for CHAI.
How did UMass Memorial join the Coalition for Health AI (CHAI)?
The journey for UMass Memorial to join CHAI started in 2018, when the Department of Radiology created an AI Steering Committee to evaluate software requests, recognizing the need for a structured framework.
Radiology really has been a ground zero for health AI tools, making the department a natural fit to serve as a hub for evaluating AI models across the system. Our radiologists were being heavily marketed to by vendors for dozens of new tools, and we did not have any type of framework for evaluating these products, or a strategy for incorporating this software into our workflows. By 2023, as the institution sought comprehensive AI governance, the radiology department’s leadership, already familiar with CHAI’s work, used the CHAI framework to develop a system-wide AI evaluation and monitoring group, and is now involved in the applied model card work.
Why has radiology been at the forefront of AI implementation in healthcare?
Radiology has been one of the easiest departments for AI to progress in because we’re already very tech-heavy and used to rapid technological change. We’re accustomed to evaluating new technologies in terms of their impact on workflow and efficiency. Bringing AI to other subspecialties that aren’t used to such rapid technological change can be more challenging.
How have CHAI’s model cards been useful to your organization?
At UMass Memorial, the AI governance process has evolved to include a broader range of members, such as legal experts and regulatory compliance representatives, necessitating a standardized approach to evaluating AI tools. CHAI’s Applied Model Cards play a crucial role in this process by consolidating information in an easily digestible format, facilitating product comparisons, and providing standardized data that meets the needs of various stakeholders, from radiologists to legal teams. Practical experience at UMass Memorial has shown that using model cards leads to more productive and organized discussions during evaluations, in contrast to working with vendors lacking model cards, which resulted in less effective communication.
What value has CHAI brought to UMass Memorial as an institution?
CHAI provides consensus guidelines that help us establish a ground reference for AI governance processes at an institutional level. This guidance has been particularly valuable as UMass Memorial expanded its AI governance from radiology to the entire healthcare system. Model cards have been extremely helpful in standardizing information and facilitating comparisons between AI products, allowing different team members to focus on the sections most relevant to their expertise. We’ve found discussions using model cards to be more organized and productive compared to evaluations without them.
What future developments are you looking forward to?
I’m particularly interested in CHAI’s upcoming guidance on evaluating generative AI tools in
healthcare. This is a rapidly evolving area where we currently lack standardized evaluation
processes. Additionally, the potential development of regional AI assurance labs could be
valuable in consolidating expertise and standardizing evaluation workflows.
Media Inquiries: [email protected]